The quality of economic forecasts tends to deteriorate during times of stress such as the COVID-19 pandemic, raising questions about how to improve forecasts during exceptional times. One method of forecasting that has received less attention is refining model-based forecasts with judgmental adjustment, or hybrid forecasting. Judgmental adjustment is the process of incorporating information from outside a model into a forecast or adjusting a forecast subjectively. Hybrid forecasts could be particularly useful during extraordinary times such as the COVID-19 pandemic, as models that do not incorporate judgment may be less able to adapt to a rapidly changing environment.
In this article, Thomas Cook, Amaze Lusompa, and Johannes Matschke explore how hybrid forecasts performed relative to pure model-based forecasts during the COVID-19 pandemic, using forecasts for imports and exports as a test. They find that hybrid models improved import and export forecasts during the trough of the pandemic but did not materially improve forecasts during the pandemic recovery or over longer forecasting horizons. They conclude that hybrid forecasts are mainly helpful for near-term forecasts during extraordinary circumstances.
Introduction
To achieve its goals of maximum employment and price stability, the Federal Open Market Committee (FOMC) needs both accurate assessments of the current state of the economy and reliable forecasts of its plausible path. However, during times of stress, such as the Great Recession or the COVID-19 pandemic, the quality of forecasts tends to deteriorate. This deterioration has raised questions about what can be done to improve forecasts during exceptional times, with several papers discussing different model-based methods as potential improvements.
One method that has received less attention is refining model-based forecasts with judgmental adjustment, or hybrid forecasting. Judgmental adjustment is the process of incorporating information from outside a model into a forecast or adjusting a forecast subjectively. This adjustment could be including qualitative information that is not easily quantified (such as developments in the news), incorporating conditions not yet captured by the model (such as a new policy being announced), or identifying and discounting outliers based on expert opinion and years of experience studying data. Hybrid forecasts could be particularly useful during extraordinary times such as the COVID-19 pandemic, as models that do not incorporate judgment may be less able to adapt to a rapidly changing environment.
In this article, we explore to what degree hybrid forecasts performed better than pure model-based forecasts during the COVID-19 pandemic, using forecasts for imports and exports as a test. We find that hybrid models improved import and export forecasts during the trough of the pandemic but did not materially improve forecasts during the pandemic recovery or over longer forecasting horizons. We conclude that hybrid forecasts are mainly helpful for near-term forecasts during extraordinary circumstances.
Section I explains the value of forecasting imports and exports. Section II describes and compares model-based, judgment, and hybrid methods. Section III compares the performance of different forecasting models as well as private-sector forecasts of U.S. imports and exports during the pandemic.
I. The Value of Forecasting Imports and Exports
Imports and exports make significant contributions to GDP, so predicting how they may change in the future can be valuable to policymakers. Chart 1 plots the annualized contribution from net exports (exports less imports) toward real GDP growth for every quarter over the last two decades—that is, the percentage point change in real GDP growth directly linked to trade. On average, U.S. imports exceed U.S. exports, so the contribution is negative. However, as the chart shows, the contribution is also highly variable. For example, the 2008–10 global financial crisis triggered a recession in the United States and led to a larger decline in imports relative to exports. As a result, the contribution from net exports to GDP increased to about 2 percentage points in the first half of 2009. However, as the U.S. economy started to recover, imports grew relative to exports and the contribution from trade turned negative. The COVID-19 pandemic and recovery marked another period of import/export volatility. During the COVID-19 pandemic, the contribution from net exports reached −2.6 percentage points (2020:Q3). In other words, real GDP growth would have been 2.6 percentage points higher if net exports were zero—a significant number considering that real GDP growth in the United States averages around 2 percent in the long run. However, during the pandemic recovery, exports increased relative to imports, and the net contribution reached 2.6 percentage points in 2022:Q3. As Chart 1 shows, imports and exports become particularly volatile when the United States enters and exits a recession.
Chart 1: Contribution of Net Exports to Real GDP Growth
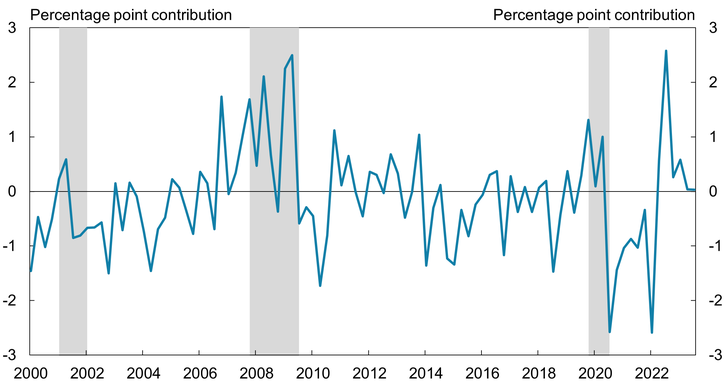
Sources: U.S. Bureau of Economic Analysis and National Bureau of Economic Research (both accessed via Haver Analytics).
Although net exports illustrate the importance of trade for GDP, we forecast imports and exports separately in this paper for a couple of reasons. First, disaggregating components can lead to better forecasting because the aggregate may mask important information. During the COVID-19 pandemic, for example, breakdowns in supply chains affected imports and exports differently due to variations in the composition of the goods being traded, the volume of trade, and trading partners’ lockdown policies. Second, imports and exports can each be informative about the economy in their own right. For example, changes in imports may be informative about domestic demand, while changes in exports may be informative about global demand.
II. Model-Based, Judgment, and Hybrid Forecasting
Given the importance of imports and exports to the U.S. economy, researchers monitor them closely and have developed several approaches to forecasting these variables. In general, forecasters use one of three methods, each with its own pros and cons: model-based forecasting, judgmental forecasting, or hybrid forecasting.
Model-based forecasts
Some of the most popular forecasting models are time-varying parameter models, which are explained in more detail in Lusompa and Sattiraju (2022). In short, time-varying parameter models mitigate parameter instability by allowing the parameters of the model to change in each time period in the sample. Intuitively, time-varying parameter models discount information over time, giving more weight to recent information about a particular economic variable than past information for any given period.
A pure model-based approach to forecasting has advantages and drawbacks. One advantage is that because model-based forecasts are more objective, they are also more reproducible with the same model and data, meaning researchers can easily communicate how they came up with a forecast. In addition, models can handle more complex data and can avoid cognitive errors that could affect human calculations. However, these advantages have trade-offs. If data are limited, the model will not perform as well. Moreover, the model may not adapt quickly enough to reflect changes occurring in real time.
Judgment
Forecasters often use judgment when data are limited or uncertainty is high. For example, during the 2008–10 global financial crisis, when uncertainty was extremely high, judgment improved forecasts of key macroeconomic variables in the UK (Galvão, Garratt, and Mitchell 2021).
Because judgment is subjective, it can also be idiosyncratic to the forecaster or forecast group; however, forecasters use a few general methods to construct their forecasts. The Delphi method, for example, aggregates forecasts from a panel of experts who each use judgment to forecast a variable of interest. This method may also require the experts to include justifications for their forecasts or allow them to update their initial forecasts after viewing the cross section of forecasts. Structured analogies are another method used to construct judgmental forecasts. For example, during the COVID-19 pandemic, many forecasters used the Great Recession as an analogy when predicting the effects of public policy, as the magnitude of the event made it seem a reasonable benchmark. A third method is scenario forecasting, which involves assigning probabilities to potential scenarios and aggregating them to get a forecast.
Some advantages of judgmental forecasting include being able to incorporate qualitative factors that are not easily quantified, being able to adjust to changing conditions that are not captured by pure models, and being able to incorporate dynamics or relationships of variables that have not occurred in past data.
However, pure judgmental forecasting also has several drawbacks. Social factors such as groupthink that affect the quality and diversity of opinions can affect forecast accuracy. For example, many economists believe there is a “Fed information effect,” in which forecasters believe the Federal Reserve knows more than they do and adjust their forecasts in response to the Fed’s forecasts (see Bauer and Swanson 2023 and references therein). This effect, in turn, leads to a lack of diversity in opinions and potentially inaccurate forecasts. A forecaster’s mood may also influence the forecast to be more optimistic or pessimistic: for example, if a forecaster sees their 401(k) tank or skyrocket, they may predict the overall stock market relatively more inaccurately.
In addition, judgmental forecasts are subject to bias, making them more difficult to reproduce. Anchoring bias occurs when a forecaster is not adapting quickly enough to new information, while overreaction bias occurs when the forecaster adapts too quickly to new information. Because no one knows the future, no one knows how reactive to be. In a pure model-based approach, the model determines how adaptive to be and is consistent across researchers using the same data and model. However, with judgmental forecasts, no two forecasters are likely to have the exact same forecast. These inconsistencies make judgmental forecasts difficult to reproduce.
Hybrid models
Hybrid models, which are the predominant method used by private-sector forecasters, try to maximize the benefits of pure model-based and pure judgmental forecasts while mitigating their risks and drawbacks (Stark 2013). In hybrid forecasting, a forecaster essentially uses a baseline model (or set of models) to generate predictions, and then adjusts these predictions using judgment where desired. The models help discipline human overreaction and biases, while judgment allows the forecaster to incorporate developments that are important but hard to quantify.
Several forecasters used hybrid methods during the COVID-19 pandemic. For example, some forecasters were encouraged by better-than-expected news on vaccine development in the second half of 2020 and made upward revisions to their otherwise model-based GDP forecasts in anticipation of the economy returning to normal sooner than expected. Similarly, when stimulus negotiations were in the news and showing promise, some forecasters adjusted their forecasts to reflect stimulus offsets to some of their assumed pandemic-driven weakness in the first half of 2021. However, despite this use during the pandemic, the performance of hybrid forecasts has not been analyzed relative to other methods. Are forecasters better off focusing on more sophisticated models during times of stress, or should they incorporate more judgmental adjustment—as most private-sector forecasters do?
III. Estimating the Performance of Forecasting Models during the Pandemic
To determine whether judgmental adjustment improves pure model-based forecasts, we compare the performance of the average of three private-sector forecasts (which proxy for hybrid models) to three different pure models._ The three pure models are the time-varying parameter model from Stock and Watson (2007), which estimates a time-varying mean and includes no other predictors; the model from Carriero and others (2022), which is a moderate-sized dimensional model that incorporates stochastic volatility and shrinkage and is designed to handle outliers; and the model from Koop and Korobilis (2023), which incorporates time-varying parameters and many predictors._ These models performed well for many macroeconomic and financial variables prior to the pandemic. Additional details on each of these models as well as their implementation are available in the appendix.
To assess the pure model-based forecasts’ accuracy in forecasting both short-term and longer-term imports and exports, we examine both one-quarter-ahead and one-year-ahead forecasts. We begin our one-quarter-ahead forecasts in 2019:Q1, one year before the start of the pandemic, and forecast imports and exports for the next quarter based on information known up until the previous quarter. For example, our one-quarter-ahead forecast for 2020:Q2 imports is based on data up until 2020:Q1. Similarly, our one-year-ahead forecast is based on information known up until the previous year. For this reason, we begin our one-year-ahead forecast in 2020:Q1. All forecasts end in 2023:Q3.
To compare the private-sector forecasts to the forecasting models, we compare the one-quarter-ahead forecast of the model to the private forecast for the current quarter. This approach allows us to more closely match the data that would be available for forecasting in practice. For example, in the eighth week of Q1, the best forecast of Q1 we could get from a model would be the one-quarter-ahead forecast using data through Q4 of the previous year. At the same time, the private forecasts would be available for Q1, presumably using sub-quarter data or judgment from Q1. Thus, comparing a one-quarter-ahead forecast from a time-varying parameter model to a current-quarter forecast from the private sector would be the most informative indicator of performance._
To judge the forecasting performance of the models and private-sector forecasts, we compare their mean absolute errors (MAE). The MAE quantifies how much a forecast deviates from the data, with smaller MAEs indicating better forecast performance. In addition, the MAE is less sensitive to the influence of outliers, in that an inaccurate prediction in one period will not have outsized influence on the forecast’s MAE relative to an accurate prediction. This quality makes MAEs especially useful during extreme circumstances such as the pandemic, which can have extraordinarily large outliers relative to normal times.
Chart 2 shows the MAE of the average private-sector forecast in light blue, the Koop and Korobilis (2023) model in purple, the Carriero and others (2022) model in green, and the Stock and Watson (2007) model in dark blue. The left half of Chart 2 shows that over our sample, the average private-sector forecasts have lower MAEs than the pure models for one-quarter-ahead import and export forecasts. A MAE of 10 means that on average, the forecast error was off by 10 percentage points (in absolute value). Thus, for the one-quarter-ahead forecasts of imports and exports, private-sector forecasts were off from the realized outcome by about 10 percentage points, while model-based forecasts were off, on average, by 15 percentage points—significant errors given that import and export growth rates are generally below 10 percent. In other words, the one-quarter-ahead private-sector forecasts outperform the pure model-based forecasts by a wide margin.
Chart 2: Mean Absolute Error of Import and Export Forecasts
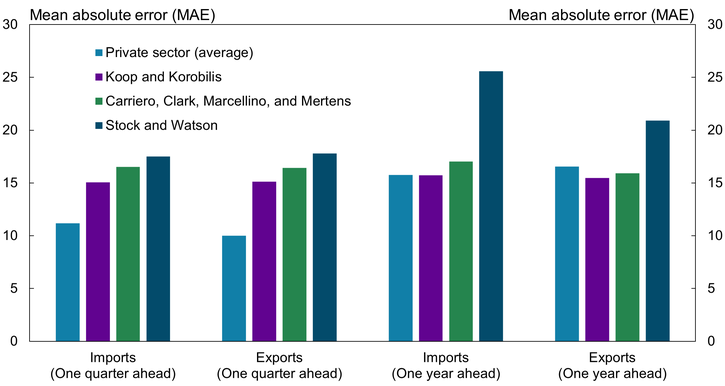
Sources: J.P. Morgan, S&P Global Market Intelligence (IHS Markit), Barclays, Koop and Korobilis (2023), Carriero and others (2022), Stock and Watson (2007), and authors’ calculations.
However, when forecasting just one year ahead, the results change substantially. The right half of Chart 2 shows that with the exception of the Stock and Watson (2007) model (dark blue), the pure models are competitive with private-sector forecasts for one-year-ahead forecasting. For exports, the Koop and Korobilis (2023) model (purple) and the Carriero and others (2022) model (green) slightly outperform the average private-sector forecast. For imports, the average private-sector forecast ties the Koop and Korobilis (2023) model and only slightly outperforms the Carriero and others (2022) model. Unsurprisingly, the MAEs for the private-sector forecast and the Stock and Watson (2007) model forecasts were more accurate for the one-quarter-ahead forecasts than they were for the one-year-ahead forecasts, as uncertainty is higher for outcomes further in the future. Interestingly, the MAEs for the Koop and Korobilis (2023) and Carriero and others (2022) model-based forecasts were about the same regardless of the horizon or the variable being forecast.
Overall, although the private-sector forecasts beat the pure models at the one-quarter horizon and do as well at the one-year horizon, they may not be uniformly preferred based solely on their MAEs. The MAE measures accuracy over the entire forecast period, so it may obscure periods when one approach does better than another; for any individual period, the pure models may perform better or worse than private-sector forecasts. To show how the performance of these forecasts has evolved over time, Chart 3 compares forecast errors—the difference between the actual and predicted values of imports and exports—over the past few years. Values closer to zero indicate a smaller forecast error and therefore better performance. Panel A of Chart 3 shows that no one model or method dominates for the one-quarter-ahead forecasts. Similarly, Panel B of Chart 3 shows that no one model or method dominates for the one-year-ahead forecasts. The main driver of the superior performance in the average private-sector forecast is driven by big misses by the pure models in the height of the pandemic-driven economic uncertainty (2020:Q1–2021:Q1). During this period, both the average private-sector forecasts and the pure model forecasts missed by a lot, though the private-sector forecasts missed by much less. At every point before (in 2019) and after (2021:Q2 and on), the pure model forecast errors are close (and sometimes better) than the average private-sector forecast.
Chart 3: Forecast Errors for Imports and Exports
Panel A: One-Quarter-Ahead Forecasts
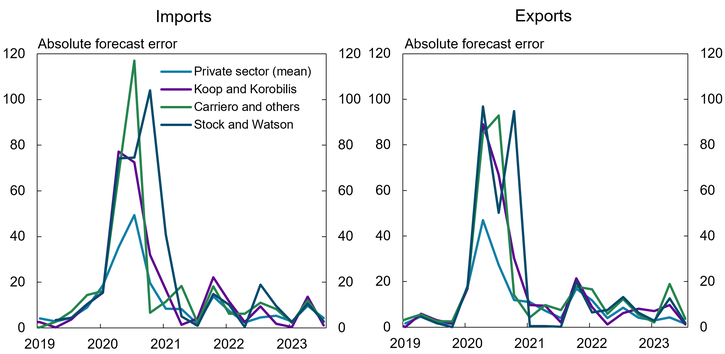
Panel B: One-Year-Ahead Forecasts
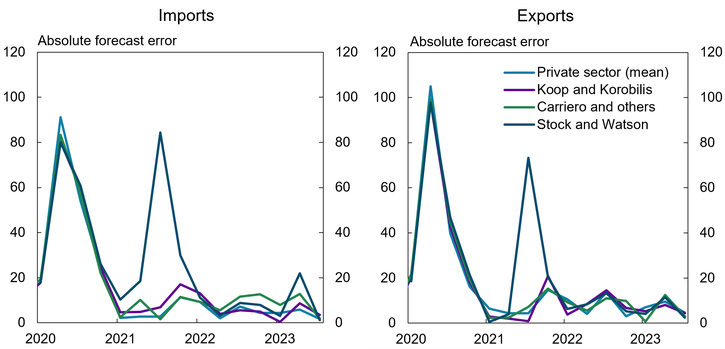
Sources: J.P. Morgan, S&P Global Market Intelligence (IHS Markit), Barclays, Koop and Korobilis (2023), Carriero and others (2022), Stock and Watson (2007), and authors’ calculations.
The Stock and Watson (2007) model stands out in this exercise, as its performance is worse than both the other models and the average private-sector forecast, particularly for the one-year horizon. This poor performance may be due to a couple of reasons. First, the Stock and Watson (2007) model estimates only a time-varying mean and includes no other predictors. Although this approach works for many variables, in this case, additional predictors may have been able to significantly improve import and export forecasts. Second, because the model only estimates a time-varying mean, it does not account for dynamics when forecasting. When forecasting one quarter ahead (for example, for 2020:Q3), the forecast is simply the time-varying mean estimate from the previous quarter (2020:Q2). When forecasting one year ahead (for example, for 2021:Q3), the forecast is simply the time-varying mean estimate from the previous year (2020:Q2). Because the time-varying mean adapted to the COVID-19 outliers, forecasts were inaccurate a year after the outlier occurred for the one-year-ahead forecast.
These results offer a few potential takeaways. First, although additional information may have improved import or export forecasts of the pure models during the pandemic, pure models included the wrong information. Macroeconomic forecasting models in general use macroeconomic and financial variables. During the COVID-19 pandemic, however, standard macro and financial variables may have been less useful in forecasting imports and exports due to the unique combination of strong demand and persistent supply shocks. Instead, the judgmental adjustment used by private-sector forecasts, based on news reports, U.S. hospitalization rates for COVID-19, or expected shipping logjam times at U.S. ports, may have been more relevant to imports and exports and thus improved these forecasts.
Second, similar to Galvão, Garratt, and Mitchell (2021), our results suggest that judgmental adjustment may not be helpful at longer horizons but can substantially improve forecasts at shorter horizons during exceptional times. Forecasters may simply be better at subjectively predicting the short run than the medium or long term, as they are not yet able to accurately incorporate judgmental adjustments at longer horizons, whereas models are more likely to be better at accounting for the longer-run behavior between variables.
Lastly, our results also suggest that forecasters should not focus on only one model but rather continuously monitor multiple models. One way to do this systematically is by using model averaging or averaging the predictions of a set of models. Some studies have shown that model averaging or combining forecasts can outperform any one model by safeguarding against a bad forecast from a single model (Hoeting and others 1999; Faust and Wright 2013). Our results also suggest that model averaging could be a useful tool for forecasting during future extreme events.
Conclusion
In this article, we investigate whether forecasters should rely more on judgmental adjustment during times of economic turmoil. We do so by exploring whether private-sector forecasts (which we use as a proxy for hybrid models) performed better than pure model-based forecasts for imports and exports during the pandemic.
We find that hybrid model forecasts do not uniformly improve the accuracy of import or export forecasts relative to pure model-based forecasts. Hybrid forecasts performed better than pure model-based forecasts for short horizons during the trough of the pandemic but about the same outside of that period. We also find that pure model-based forecasts are competitive during normal times, suggesting that judgmental adjustment may help forecasting short horizons during uncertain times but may not make a material improvement during normal times or longer horizons.
Appendix
Model specifications
All models use four lags except for Stock and Watson (2007), which has no lags. For Stock and Watson (2007), we use the non-centered parameterization and priors from Chan (2017). For Carriero and others (2022) and Koop and Korobilis (2023), we used the same priors as in the papers. All the samples start approximately around 1980, give or take a couple years due to data availability.
Alternative timing of private-sector forecasts
To compare private-sector forecasts to the forecasting models, we compare the one-quarter-ahead forecast of the model to the private forecast for the current quarter. Instead, we could compare the one-quarter-ahead forecast from the models with the one-quarter-ahead private-sector forecasts generated late in the previous quarter. However, this builds a substantial and artificial information disadvantage into the comparison. To compare Q1 forecasts, we would need to take the one-quarter-ahead private forecast of Q1 generated at some late date in Q4 of the previous year, as private forecasters would only have access to quarterly data through Q3. At the same time, we would not be able to generate the one-quarter-ahead model-based forecast for Q1 until some point beyond roughly the halfway point of Q1, because import and export numbers are released at a two-month lag, and that forecast would be informed by quarterly data through Q4. If the model-based forecast turns out to have a lower error in this comparison, we cannot be sure whether the result is capturing the improvement in forecasts from using a particular forecast method or from using more up-to-date data.
Endnotes
-
1
We proxy for hybrid models using the average of private-sector forecasts, as most private-sector forecasters use judgment of some kind (Stark 2013 and Galvão, Garratt, and Mitchell 2021). The private-sector forecasts are from Barclays, JP Morgan, and IHS Markit.
-
2
In the Carriero and others (2022) model, only the covariance matrix is time-varying.
-
3
Lags in data releases give the private-sector forecast an informational advantage over the model-based forecasts, which depend solely on these data. Even if a forecaster accounts for these lags, private-sector forecasts still outperform model-based forecasts, but by a significantly smaller margin. See the appendix for further discussion.
Publication information: Vol. 109, no. 5
DOI: 10.18651/ER/v109n5CookLusompaMatschke
References
Bauer, Michael D., and Eric T. Swanson. 2023. “An Alternative Explanation for the ‘Fed Information Effect.’” American Economic Review, vol. 113, no. 3, pp. 664–700. Available at External Linkhttps://doi.org/10.1257/aer.20201220
Carriero, Andrea, Todd E. Clark, Massimiliano Marcellino, and Elmar Mertens. 2022. “Addressing COVID-19 Outliers in BVARs with Stochastic Volatility.” Review of Economics and Statistics, Forthcoming. Available at External Linkhttps://doi.org/10.1162/rest_a_01213
Chan, Joshua C. C. 2017. “Specification Tests for Time-Varying Parameter Models with Stochastic Volatility.” Econometric Reviews, vol. 37, no. 8, pp. 807–823. Available at External Linkhttps://doi.org/10.1080/07474938.2016.1167948
Faust, Jon, and Jonathan H. Wright. 2013. “Forecasting Inflation,” in Graham Elliott and Allan Timmermann, eds., Handbook of Forecasting: Volume 2, pp. 2–56. Amsterdam: Elsevier.
Galvão, Ana Beatriz, Anthony Garratt, and James Mitchell. 2021. “Does Judgment Improve Macroeconomic Density Forecasts?” International Journal of Forecasting, vol. 37, no. 3, pp. 1247–1260. Available at External Linkhttps://doi.org/10.1016/j.ijforecast.2021.02.007
Hoeting, Jennifer A., David Madigan, Adrian E. Raftery, and Chris T. Volinsky. 1999. “Bayesian Model Averaging: A Tutorial.” Statistical Science, vol. 14, no. 4, pp. 382–417. Available at External Linkhttps://doi.org/10.1214/ss/1009212519
Koop, Gary, and Dimitris Korobilis. 2023. “Bayesian Dynamic Variable Selection in High Dimensions.” International Economic Review, vol. 64, no. 3, pp. 1047–1074. Available at External Linkhttps://doi.org/10.1111/iere.12623
Lusompa, Amaze, and Sai A. Sattiraju. 2022. “Cutting-Edge Methods Did Not Improve Inflation Forecasting during the COVID-19 Pandemic.” Federal Reserve Bank of Kansas City, Economic Review, vol. 107, no. 3, pp. 21–36. Available at External Linkhttps://doi.org/10.18651/ER/v107n3LusompaSattiraju
Stark, Tom. 2013. “SPF Panelists’ Forecasting Methods: A Note on the Aggregate Results of a November 2009 Special Survey.” Federal Reserve Bank of Philadelphia.
Stock, James H., and Mark W. Watson. 2007. “Why Has U.S. Inflation Become Harder to Forecast?” Journal of Money Credit and Banking, vol. 39, pp. 3–33. Available at External Linkhttps://doi.org/10.1111/j.1538-4616.2007.00014.x